Recently, the legendary figure in the chip industry, processor design guru, and current CEO of Tenstorrent, Jim Keller, stated in an interview that Nvidia has not served many markets well, thus providing opportunities for Tenstorrent and other emerging AI processor development companies.
Jim Keller has held positions at several major companies, including AMD, Intel, Apple, and Tesla. From 1998 to 1999, Jim Keller led the development of the K7/K8 architecture that supported the Athlon series of processors at AMD. From 2008 to 2012, he led the development of the A4 and A5 processors at Apple. From 2012 to 2015, he led the K12 Arm project and the Zen architecture project at AMD. From 2016 to 2018, he developed the FSD autonomous driving chip at Tesla, and from 2018 to 2020, he participated in a mysterious project at Intel.
Now, Jim Keller is leading the development of AI processors at Tenstorrent, which can provide a cost-effective alternative to Nvidia's expensive GPUs. Nvidia's GPUs are priced at $20,000 to $30,000 each or more. Tenstorrent claims that its Galaxy system is three times more efficient than Nvidia's DGX, with a cost reduction of 33%. Providing product alternatives for high-performance AI application processors is part of Tenstorrent's work, but not all of it. The company's business purpose is to serve the market pain points that Nvidia has not addressed, especially in the field of edge computing.
Advertisement
01
Edge Computing AI Status Enhancement
With the continuous increase of massive data and the increasing demand for real-time and security requirements of computing and storage systems, data centers can no longer meet the needs of the market and customers. The market requires related hardware and software system providers to find faster ways to serve customers, to improve operational efficiency and reduce costs. Edge-to-cloud solutions that run AI workloads at the edge help to meet this demand, placing computing power at the network edge close to the point of data creation, which is crucial for applications that require near real-time performance, processing algorithms and data on local devices instead of transmitting these workloads to the cloud or data center.
With the development of 5G and the Internet of Things, the application prospects of AI chips in the field of edge computing are very broad, such as autonomous driving vehicles, smart cities, and other scenarios, all of which require real-time AI inference on terminal devices. To this end, many manufacturers have launched AI chips specifically for edge inference.
In the manufacturing industry, locally running AI models can quickly respond to data from sensors and cameras to perform important tasks. For example, car manufacturers use computer vision to scan assembly lines to identify potential defects in vehicles before they leave the factory. In such applications, very low latency and always-on requirements make it impractical to transmit data back and forth across the entire network. Even a small amount of latency can affect product quality. In addition, low-power devices cannot handle large AI workloads, such as the models that rely on training computer vision systems. The overall edge-to-cloud solution combines the advantages of both ends, with the backend cloud providing scalability and processing power for complex AI workloads, and the front-end edge devices closely integrating data and analysis to minimize latency.
Taking the Arduino low-power edge device as an example, the cost of many such devices is less than $100, and users can combine several or thousands of devices running machine learning models. For example, an agricultural company uses the Arduino solution to maximize crop yield, involving sensors that provide edge devices with data such as soil moisture and wind conditions to determine the amount of water needed by the crops. This technology can help farmers avoid overwatering and reduce the operating costs of electric water pumps.For instance, a manufacturer reliant on precision lathes combines sensors with Arduino devices to detect anomalies such as minuscule vibrations, which are indicative of potential equipment malfunctions. For businesses, regular maintenance is more cost-effective than dealing with unexpected breakdowns that halt production.
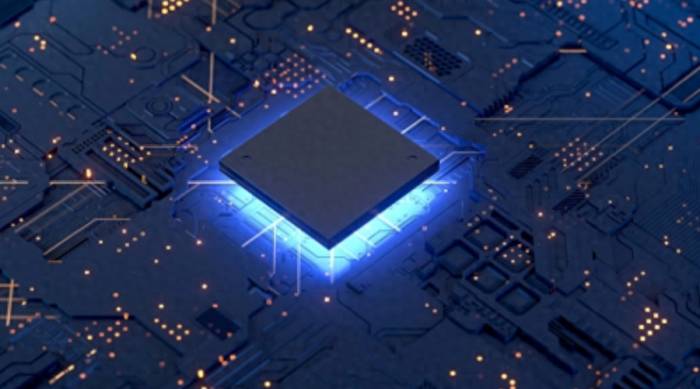
These applications demonstrate the value and role of edge computing. Given the current development of applications, the demand for such uses is increasing, as is the need for intelligent control, which is where the value of edge AI lies. Companies like Nvidia, whose high-performance chips such as GPUs are primarily focused on the AI servers in cloud computing and data center markets, pay little attention to the edge AI market. This presents opportunities for AI chip companies like Tenstorrent.
02
More AI Chip Companies Challenge Nvidia
As various players rush to invest, the AI chip market is becoming a hub of diverse competition. According to statistics, the number of global AI chip startups exceeded 80 in 2019, with a total funding amount exceeding $3.5 billion. Research institutions estimate that by 2025, ASICs will account for 43% of the AI chip market, GPUs 29%, FPGAs 19%, and CPUs 9%.
A batch of AI chip startups is on the rise, with Tenstorrent, mentioned earlier, being a typical representative; Cerebras Systems has created the largest chip ever, the WSE (Wafer Scale Engine), equipped with 1.2 trillion transistors, bringing AI computation to an unprecedented scale; the star company Groq, founded by former Google engineers, focuses on creating low-power processors for AI inference.
An introduction to Tenstorrent's technology and products is in order, as it places special emphasis on low power consumption, making it more suitable for edge AI applications. According to Nikkei News, Tenstorrent is expected to release its second-generation multi-purpose AI processor by the end of 2024, but the name of the processor has not been disclosed. According to the company's roadmap released in the fall of 2023, it plans to release its Black Hole standalone AI processor and Quasar low-power, low-cost chip.
In the early years, when Jim Keller served as CTO of Tenstorrent, he was very optimistic about the low-power RISC-V architecture, and his team developed the Ascalon CPU based on this. It is reported that the company's new generation of Black Hole AI chips is developed based on the SiFive X280 RISC-V core design.
Tenstorrent stated that one of the important reasons for the high efficiency and lower cost of the upcoming processor is the avoidance of high-bandwidth memory (HBM), and the switch to GDDR6, which is very well-suited for entry-level AI processors designed for AI inference. That is to say, the company's AI chip architecture consumes less memory bandwidth than its competitors, thus reducing costs.
Although Tenstorrent has not yet captured a significant share of the AI processor market, the company's cost-effective and scalable AI solutions can meet a variety of application needs that Nvidia cannot reach. Not only Tenstorrent, but several other new AI chip companies will also launch similar application AI chip products in the next few quarters. In summary, instead of competing head-on with Nvidia, more and more new entrants in the AI market are focusing on markets that have not been occupied by the "green team".Innovation in AI chips has been ongoing, with significant room for optimization not only in terms of computational power but also in architecture, power consumption, and integration. For instance, through advanced packaging technologies, multiple AI chips can be closely integrated, significantly enhancing system bandwidth and energy efficiency. AI-specific memory technologies, such as HBM (High Bandwidth Memory) and compressed memory, will also see broader applications.
03
Challenging NVIDIA's Ecosystem
In addition to chip technology innovation, the construction of an AI ecosystem is equally important. NVIDIA's CUDA platform has developed over the years to form a vast developer community and a rich array of software resources, which is a crucial guarantee of its competitiveness.
Other manufacturers are also following suit, building ecosystems around their own AI chips to win the support of developers. Google has launched the TensorFlow deep learning framework based on TPU and open-sourced the related code; AMD has acquired Xilinx; Intel has introduced the OneAPI development tool suite, attempting to unify the programming interfaces for CPUs, GPUs, and AI accelerators.
Arm, Intel, Qualcomm, Samsung, and others have collaborated to establish the Unified Acceleration Foundation (UAF), one of whose goals is to replace NVIDIA's solutions.
In AI systems, chip interconnect technology is crucial, especially data transfer bandwidth, which plays an important role in the performance of the system. NVIDIA has been building its own ecosystem in this area. The company's latest Blackwell GPU will use the newly launched NVLink standard protocol for multi-chip and network interconnects. In data center networks, NVIDIA uses its own InfiniBand bus.
Jim Keller is not fond of NVIDIA's closed ecosystem. He is a staunch supporter of open technology and strongly dislikes closed technologies.
Jim Keller proposed that NVIDIA should not use the proprietary NVLink standard protocol and should switch to the open Ethernet standard. He also believes that in data center networks, NVIDIA should not use InfiniBand but should switch to Ethernet as well. Although InfiniBand has characteristics of low latency and high bandwidth (up to 200Gb/s), Ethernet can achieve 400Gb/s or even 800Gb/s.
In fact, giants such as AMD, Broadcom, Intel, Meta, Microsoft, and Oracle are collaborating to develop the next generation of ultra-high-speed Ethernet (Ultra Ethernet), which has higher throughput and is more suitable for AI and HPC (High-Performance Computing) applications.So, can the new Ethernet technology develop and compete with Nvidia's interconnect technology?
In July 2023, several industry giants established the Ultra Accelerator Link (UALink) alliance, aiming to compete with Nvidia's InfiniBand.
AMD is contributing to UALink by working hard on a broader Infinity Fabric shared memory protocol and GPU-specific xGMI, and all other participants have agreed to use the Infinity Fabric as the standard protocol for accelerator interconnection. Sachin Katti, Senior Vice President and General Manager of Intel's Networking and Edge Group, said that the "promotion group" of the Ultra Accelerator Link, composed of AMD, Broadcom, Cisco Systems, Google, Hewlett Packard Enterprise, Intel, Meta Platforms, and Microsoft, is considering using the first layer of Ethernet transmission protocol and Infinity Fabric as a way to link GPU memory to a huge shared space similar to NUMA on the CPU.
Members of the UALink alliance believe that system manufacturers will create devices using UALink and allow accelerators from many participants to be placed in these devices when customers build their Pods. You can have a pod with an AMD GPU, a pod with an Intel GPU, and another pod with some custom accelerators from other manufacturers. The device can achieve universality in server design, just like the Open Accelerator Module (OAM) specification released by Meta Platforms and Microsoft, with universality of accelerator slots on the system board.
According to IDC, there are already enough 200Gb/s and 400Gb/s network constructions in hyperscale enterprises, cloud builders, HPC centers, and large enterprises, and the InfiniBand and Ethernet markets can grow simultaneously.
Ethernet is ubiquitous - at the edge and in the data center - which is different from InfiniBand, which is specifically used in the data center. IDC said that in the third quarter of 2023, the sales of data center Ethernet switches increased by 7.2% year-on-year.
From the third quarter of 2022 to the third quarter of 2023, the market size of data center Ethernet switches is about 20 billion US dollars. If the switch accounts for half of the InfiniBand revenue, then the scale of data center Ethernet switches is still about 7 times larger than that of InfiniBand switches. Moreover, more and more AI clusters are migrating to Ethernet, which is eroding the market share of InfiniBand.
IDC said that in the non-data center part of the Ethernet switch market, the sales growth is faster, with an increase of 22.2% in the third quarter of 2023, and a total increase of 36.5% in the first three quarters, because many companies have upgraded their campus networks.In the third quarter of 2023, the market size of Ethernet switches for data centers, campuses, and edge sides reached 11.7 billion US dollars, a year-on-year increase of 15.8%. The accompanying Ethernet router market declined by 9.4%, which is not surprising, as routers are increasingly built with commercial chips that include both switching and routing functions.
In data centers, the sales of 200Gb/s and 400Gb/s Ethernet switches increased by 44% year-on-year, and the port shipments increased by 63.9% year-on-year. The sales of 100Gb/s Ethernet switches for data centers, edge sides, and campuses increased by 6%.
Conclusion
NVIDIA's advantage in cloud computing and data center AI systems is very obvious. Whether it is a chip industry giant or a startup, it is very difficult to compete with NVIDIA in this field, especially for startups. In the past few years, several small companies that took NVIDIA's GPU as a competitor and followed the same track did not fare well, with some even on the brink of bankruptcy. Only big companies like AMD and Intel can compete with NVIDIA in the same field.
The cloud computing and data center AI track is not easy to catch up with, so focus on the low power consumption and low cost edge side application market, which is also a huge market with broad development space, and there are not many chips on the market that are suitable and applicable at present. In this situation, whoever takes the initiative early and quickly produces practical products will gain an advantage in future competition.
In addition to AI chips, interconnection technology and standards are also a very promising investment direction, and there are opportunities in both data centers and edge sides. NVIDIA's interconnection and bus technology cannot cover all aspects. As AI technology continues to penetrate various industries, as well as various performance, power consumption, and cost application levels, the development space for chip and system interconnection will become larger and larger, and companies of all sizes are eager to try their best.
Leave a Reply