The "computing power" related industries have been booming recently, and the construction of smart computing centers is also blossoming everywhere.
Entering 2024, there have been Wuchang Smart Computing Center, China Mobile Smart Computing Center (Qingdao), South China Data Valley Smart Computing Center, Zhengzhou Artificial Intelligence Computing Center, and Boda Data Shenzhen Qianhai Smart Computing Center, etc., which have started construction or put into use one after another.
According to incomplete statistics, there are already more than 30 cities across the country that are building or proposing to build smart computing centers, with an investment scale of more than ten billion yuan.
What exactly is a smart computing center? What is a smart computing center mainly used for? What are the characteristics of smart computing centers?
01
What is a Smart Computing Center?
According to the definition in the "Action Plan for High-Quality Development of Computing Power Infrastructure," a smart computing center refers to a facility that uses a large-scale heterogeneous computing power resource, including general computing power (CPU) and intelligent computing power (GPU, FPGA, ASIC, etc.), mainly to provide the required computing power, data, and algorithms for artificial intelligence applications (such as the development of artificial intelligence deep learning models, model training, and model inference, etc.).
Advertisement
It can also be said that a smart computing center is a data center mainly for artificial intelligence computing tasks.Data centers typically include three categories, in addition to the intelligent computing center, the other two are the general computing center, which is mainly for general computing tasks, and the supercomputing center, which is mainly for supercomputing tasks.
The year 2023 is an important turning point for the development of artificial intelligence. AIGC technology has made breakthrough progress, and new businesses such as large model training and large model application are rapidly emerging. As the carrier of intelligent computing power, data centers have also evolved from data rooms and general computing centers to the current stage of supercomputing centers and intelligent computing centers.
What is the difference between an intelligent computing center and a general data center?
Intelligent computing centers are usually closely related to cloud computing, emphasizing the flexibility of resource control and infrastructure management. In the cloud environment, data center providers are responsible for the maintenance of hardware and some software tools, while customers own the data. In contrast, traditional local data centers require companies to manage and maintain all data resources themselves.
The essential differences lead to significant differences between the two models in terms of capital investment, resource deployment, and security.
In terms of capital investment, customers of intelligent computing centers can choose their own service model, such as public cloud, private cloud, or hybrid cloud, without the need for a large amount of hardware and software costs; while customers of traditional data centers need to invest a lot of money to purchase and maintain their own servers, networks, and storage devices.
In terms of resource deployment and security, customers of intelligent computing centers can remotely access and manage their own data and applications anytime, anywhere, through the Internet. At the same time, they can also enjoy professional security protection provided by data center providers, such as firewalls, encryption, backup, and recovery; while customers of traditional data centers are limited by office/specified locations and need to protect and manage data themselves.
In simple terms, an intelligent computing center is a data computing center specifically serving artificial intelligence, capable of providing the dedicated computing power required for AI calculations. Compared with traditional data centers, intelligent computing centers can meet more targeted needs, as well as provide larger computing volume and faster computing speed, providing AI computing power for various vertical industry scenarios such as large model training and inference, autonomous driving, and AIGC.AI Intelligence Calculation, What Kind of Chip Does It Need?
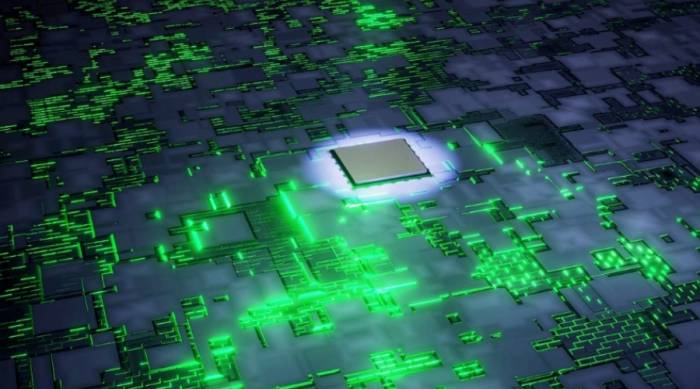
In terms of hardware selection, the hardware architecture of an intelligent calculation center differs from that of a traditional data center.
What kind of computing power chip is needed for AI intelligence calculation?
The hardware architecture of a traditional data center is relatively simple, mainly consisting of servers, storage devices, and network devices. Compared to this, the hardware architecture of an intelligent calculation center is more flexible, and different application scenarios will choose different computing nodes.
The intelligent calculation server is the main computing power hardware of the intelligent calculation center, usually adopting a heterogeneous computing architecture such as "CPU+GPU," "CPU+NPU," or "CPU+TPU" to fully leverage the advantages of different computing power chips in terms of performance, cost, and energy consumption.
GPUs, NPU, and TPU have a large number of cores and are good at parallel computing. AI algorithms involve a large number of simple matrix operation tasks, requiring strong parallel computing capabilities.
Traditional general-purpose servers, on the other hand, use CPUs as the main chip to support basic general-purpose computing such as cloud computing and edge computing.
What kind of storage chip does AI intelligence calculation need?
It's not just the difference in computing power chips; AI intelligence calculation also has higher requirements for storage chips.Firstly, it is about the amount of usage. The DRAM capacity of an intelligent computing server is typically 8 times that of a regular server, and the NAND capacity is 3 times that of a regular server. Even the number of layers of its PCB circuit boards is significantly more than that of traditional servers.
This also means that intelligent computing servers need to layout more storage chips to achieve the required performance.
As demand rises, a series of bottleneck issues have also emerged.
On the one hand, the traditional von Neumann architecture requires data to be loaded into memory, resulting in low data processing efficiency, high latency, and high power consumption; on the other hand, the memory wall problem causes the processor performance to grow much faster than the memory speed, causing a large amount of data to be transferred between the SSD and memory; in addition, the capacity and bandwidth limitations of the SSD mounted on the CPU also become performance bottlenecks.
Faced with issues such as the "memory wall" and "power consumption wall," the computing and storage architecture in the traditional computing architecture urgently needs to be upgraded, integrating storage and computing organically, with its huge potential for energy efficiency improvement, to meet the massive data storage needs of the intelligent computing era.
In response to this series of issues, the storage-computing integrated chip may be a good answer.
In addition to the difference in chips, in order to fully exert performance and ensure stable operation, AI servers have also been strengthened in terms of architecture, heat dissipation, topology, and other aspects.
04
Who is laying out these chips?
The layout of computing power chipsIn the field of GPU, GPUs excel at large-scale parallel computing. Companies such as Huawei, Tianshu Zhixin, Moore Threads, Sugon, Sui Yuan Technology, NVIDIA, Intel, and AMD have all launched relevant chips. For example, Huawei has introduced the Ascend series AI chips, including Ascend 910 and Ascend 310, which are designed specifically for AI training and inference, characterized by high performance and low power consumption. The Ascend series has been widely applied in data centers, cloud services, and edge computing, providing strong computational support for intelligent computing centers.
NVIDIA has launched several GPU products for AI training and inference, such as A100, H100, etc. Intel has also launched several AI chip products, such as the Gaudi series chips from Habana Labs, aimed at competing with NVIDIA. AMD has also laid out in the field of AI chip, launching MI series GPU and APU products.
In terms of FPGA, the combination of CPU + FPGA combines flexibility and high efficiency, adapting to the rapid changes in algorithms. Xilinx and Intel are the main players in the market, with related products including Xilinx's VIRTEX, KINTEX, ARTIX, SPARTAN product series, and Intel's Agilex product series; domestic main manufacturers include Fudan Microelectronics, Unisplendour, and Anlu Technology, etc.
In terms of ASIC, CPU + ASIC provides high-performance customized computing, suitable for specific needs. Foreign giants such as Google, Intel, and NVIDIA have successively released ASIC chips. Domestic manufacturers such as Cambricon, HiSilicon, and Horizon Robotics have also launched ASIC chips for accelerating deep neural networks.
In the aspect of NPU, NPU is a processor specifically designed for artificial intelligence and machine learning scenarios. Unlike CPUs and GPUs, NPU has been specifically optimized in hardware structure, focusing on executing AI-related computing tasks such as neural network inference. The combination of CPU's generality and NPU's specificity makes the entire system flexible to cope with various AI application scenarios and quickly adapt to changes in algorithms and models.
Currently, there are many mass-produced NPU or chips equipped with NPU modules on the market, including well-known ones such as Qualcomm Hexagon NPU and Huawei's Ascend series. It is worth noting that major manufacturers all have unique strategies in the design of chip computing cores.
In terms of TPU, TPU is a chip specifically developed by Google to accelerate the computing power of deep neural networks, more focused on processing large-scale deep learning tasks, with higher computing power and lower latency. TPU is also a type of ASIC chip.
In terms of DPU, DPU is specifically designed for data processing tasks, with a highly optimized hardware structure, suitable for computing needs in specific fields. Unlike CPUs for general computing, GPUs for accelerated computing, DPU is the third main chip in the data center. DPU products of the three international giants NVIDIA, Broadcom, and Intel occupy most of the domestic market, and manufacturers such as Xilinx, Marvell, Pensando, Fungible, Amazon, and Microsoft have also produced DPU or similar architecture products in the past 2-5 years. Domestic manufacturers include Zhongke Yushu, Xinqi Yuan, Yunbao Intelligence, Dayu Zhixin, Alibaba Cloud, etc.
Where has the domestic computing power chip come to?
At the 2024 Beijing Mobile Computing Power Network Conference, the Beijing node of China Mobile's computing power center was officially put into use, marking a new stage in the construction of China's intelligent computing centers. As the first large-scale training and pushing integrated intelligent computing center in Beijing, the project covers an area of about 57,000 square meters, with nearly 4,000 AI acceleration cards deployed, the domestication rate of AI chips reaching 33%, and the intelligent computing power scale exceeding 1000P.Recently, Zhen Yanan, the Chief Technology Officer of Beijing Beilong Super Cloud Computing Co., Ltd., the operating entity of the Beijing Super Cloud Computing Center, stated that it currently takes only about 15 days to "graft" domestic large-scale models onto domestic chips to get them running smoothly. He believes that shared computing power will be a major industry trend, and the high-end GPU computing power resources require the efforts of all parties.
In recent years, the market pattern of China's artificial intelligence computing power chips has been mainly dominated by NVIDIA, which occupies more than 80% of the market share.
Zhen Yanan said, "We are also very concerned about the development of domestic chips. It is understood that domestic self-developed large models, and even some open-source large models, are continuously being transplanted onto domestic chips. Now, from the perspective of chip usage, some models can already run smoothly, and the main areas that need to catch up are similar to high-performance GPUs."
"The entire domestication is layered, and the chip belongs to the hardware layer. In addition to this, there is also the software ecosystem. For domestic chips, whether it is the framework or the ecosystem, there is a certain nurturing cycle required." Zhen Yanan called for the ultimate application side to give enough confidence to domestic chips.
The layout of storage chips
The smart computing center needs to have characteristics such as high capacity, high reliability, and high availability in the storage area. Storage devices usually use high-performance hard drives or solid-state drives, and are equipped with redundant storage architecture to ensure the security and accessibility of data. Samsung, Micron, SK Hynix, and others have related chips that are widely used in data centers, cloud computing, and other fields, providing high-performance storage solutions for smart computing centers.
Domestic manufacturers have also achieved rapid development in the pursuit of DRAM and NAND technology in recent years.
In addition to traditional storage chips, smart computing centers also need the new type of storage mentioned above - the storage-computing integrated chip to play a greater role.
Looking at the development process of the storage-computing integration, since 2017, large factories such as NVIDIA, Microsoft, and Samsung have proposed prototypes of storage-computing integration, and domestic storage-computing integrated chip companies began to emerge in the same year.
The demand for the storage-computing integrated architecture by large factories is practical and quickly implemented, and as the technology closest to engineering implementation, near-computing has become the first choice for large factories. Large factories with rich ecosystems such as Tesla and Samsung, as well as traditional chip giants such as Intel and IBM, are all laying out near-computing.Domestic startups are focusing on in-memory computing that does not require consideration of advanced process technologies. Among them, Zhicun Technology, Yizhu Technology, Jiutian Rui Xin, and other startups are betting on PIM, CIM, and other "storage" and "computation" closer integrated storage-computing technology routes. Yizhu Technology and Qianxin Technology focus on AI high-computing scenarios such as large model computing and autonomous driving; Shanyi, Xinyi Technology, Pingxin Technology, and Zhicun Technology focus on edge small computing scenarios such as the Internet of Things, wearable devices, and smart home.
Yizhu Technology is committed to designing AI high-computing chips with an integrated storage-computing architecture, combining the memristor ReRAM and integrated storage-computing architecture for the first time, and providing a new path for the development of AI high-computing chips with higher cost-effectiveness, higher energy efficiency, and greater computing power development space through a fully digital chip design approach.
Qianxin Technology is dedicated to the research and development of high-computing integrated storage-computing chips and computing solutions in the fields of artificial intelligence and scientific computing. In 2019, it took the lead in proposing a reconfigurable integrated storage-computing technology product architecture, which can improve the computing throughput by 10-40 times compared to traditional AI chips. At present, Qianxin Technology's reconfigurable integrated storage-computing chip (prototype) has been tested or deployed in fields such as cloud computing, autonomous driving perception, image classification, and license plate recognition; its high-computing integrated storage-computing chip product prototype has also passed the internal test of domestic Internet giants for the first time.
Zhicun Technology's solution is to redesign the storage device, using the physical properties of the Flash memory storage unit, to transform and redesign the peripheral circuit of the storage array to accommodate more data, while also storing the operator in the storage device, enabling each unit to perform analog calculations and directly output the calculation results, to achieve the purpose of integrated storage-computing.
05
The proportion of intelligent computing scale exceeds 30%, and the construction of computing power is in full swing
At the beginning of July, the Tianfu Intelligent Computing Southwest Computing Center was officially put into operation in Chengdu, Sichuan. According to reports, the center will support Chengdu in building a hundred billion-level artificial intelligence core industry with computing power, and empower AI innovation in industrial manufacturing, natural science, biomedicine, and scientific research simulation experiments.
This is not an isolated case. In the past month, the Yinchuan Green Intelligent Computing Center project has started construction; Beijing Mobile has built the first large-scale training and pushing integrated intelligent computing center in Beijing, supporting the training and reasoning of hundreds of billions and trillions of high complexity and high computing demand models; the Zhengzhou Artificial Intelligence Computing Center has started construction, with a total investment of more than 1.6 billion yuan... The digital new infrastructure represented by the intelligent computing center is accelerating the construction and landing.
Data released by the National Bureau of Statistics on July 15th shows that as of the end of May, 460,000 new 5G base stations have been built nationwide; there are more than 10 intelligent computing centers planned with high-performance computer clusters, and the proportion of intelligent computing power in the total scale of computing power exceeds 30%.According to incomplete statistics from the China IDC Circle, as of May 23, 2024, there are a total of 283 intelligent computing centers in mainland China, covering all provinces, autonomous regions, and municipalities directly under the central government. Among them, there are 140 intelligent computing center projects with investment statistics, with a total investment of 436.434 billion yuan. There are 177 intelligent computing center projects with planned computing power scale statistics, with a total computing power scale reaching 369,300 PFlops.
These "intelligent computing centers" have different standards and scales, with computing power scales generally ranging from 50P, 100P, 500P, 1000P, and some even exceeding 12,000P. Although the AI wave has brought broad development prospects to intelligent computing centers, supply and demand mismatch, high prices, and redundant construction are still problems faced by China's computing power construction.
At the same time, many places have also introduced special plans, clarifying the construction goals for the next few years, and improving support measures in terms of technology, application, and funding. For example, Jiangsu released a special plan for the development of provincial-level computing infrastructure, proposing that by 2030, the total computing power in use in the province will exceed 50 EFLOPS (EFLOPS refers to the number of floating-point operations per second of 100 billion billion), and the proportion of intelligent computing power will exceed 45%; Gansu proposed to provide policy support in terms of land, municipal supporting facilities construction, talent introduction, and funding for the new type of infrastructure of the computing power network.
"The explosive development of applications such as large-scale artificial intelligence models has driven a surge in demand for intelligent computing power." Dan Zhiguang, director of the Department of Informatization and Industrial Development of the National Information Center, said that intelligent computing has developed rapidly and has become the fastest-growing type in China's computing power structure. Among them, large models are the largest demand side for intelligent computing power, accounting for nearly 60% of the demand. It is expected that by 2027, the annual compound growth rate of China's intelligent computing power scale will reach 33.9%.
Leave a Reply